Use AI to solve product problems–not PR problems, writes Intercom senior data scientist Kevin McNally.
Has artificial intelligence reached the peak of inflated expectations yet? In some circles, it’s being described as “stupid” and over-valuated. In others, AI is on its way to surpassing human intelligence in our lifetime.
We know one thing is certain: The number of companies mentioning “artificial intelligence” in earnings calls this year skyrocketed, from 6 in 2013 to 244 in 2017. Everyone from Starbucks to Mastercard is touting the benefits of AI.
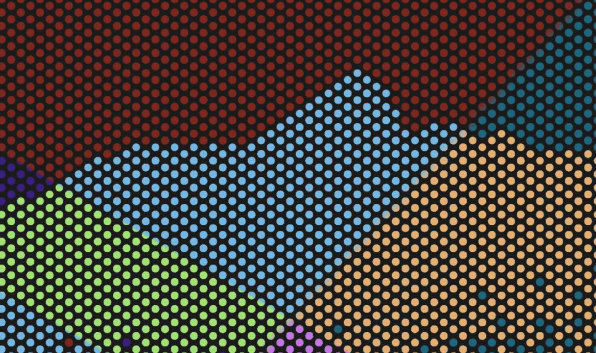
Why is there so much hype now? Much of it can likely be attributed to the following:
-
We have more data and greater computational power than ever before, giving older, well-worn concepts more horsepower.
-
We have seen innovation in techniques that take advantage of this greater power, such as deep learning.
-
Communication tools are on the rise, and as a result, companies are experimenting with AI approaches in a much higher-profile, consumer-facing interface, namely text- and voice-based messaging.
-
Technology and product advancements are reaching a point where AI can be used to augment them (e.g., IoT, driverless cars, etc.).
As a researcher embedded within a product team, it puzzles me to see so many companies pitch “X . . . with artificial intelligence!” What does that even mean for their customers? Messages like this always make me wonder whether the company is using AI as a marketing gimmick rather than solving anything of real value to the customer. In truth, AI as it stands today is rarely the most elegant solution for all your product’s problems. But with a product-first mind-set and the right circumstances, it can absolutely help you build a great product.
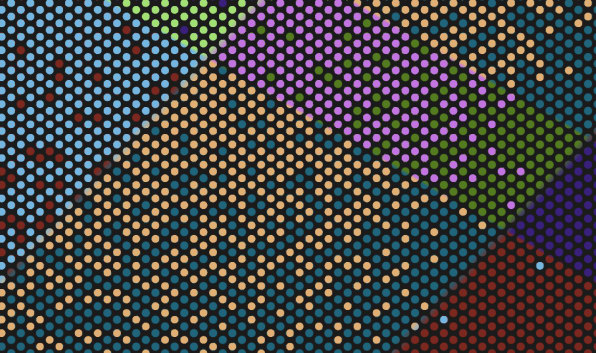
WHAT IS AI GOOD FOR TODAY?
There are many fantastic introductions to AI (this one from Andreessen Horowitz is one of my favorites), but usually when people talk about AI, they’re talking about one of two things:
- Machine learning: technologies that solve specific problems without being coded explicitly, such as self-driving cars, e-commerce chatbots, and recommendation systems. This is the only AI that’s useful today.
- Artificial general intelligence: AI that can generally engage with problems without being explicitly trained and can figure out how to solve completely new problems, like a human can. This is the science fiction, brain-plus-computer stuff that Elon Musk and Ray Kurzweil talk about. We’re not talking about general intelligence in this post.
Great, product-driven companies understand that machine learning and artificial intelligence aren’t solutions in and of themselves. They’re one solution, with strengths and weaknesses just like any other potential solution, and solve real customer pain points. Here are a few of my favorite examples:
Slack has a team called Search, Learning, and Intelligence dedicated to helping customers cut down on time-consuming work tasks, like searching for files, suggesting channels to leave or join, and catching up on discussions taking place after work hours. The team is trying to solve a massive problem: information overload. For instance, a nice new set of features called Highlights uses machine learning to surface a user’s most important messages, based on their activity across channels.
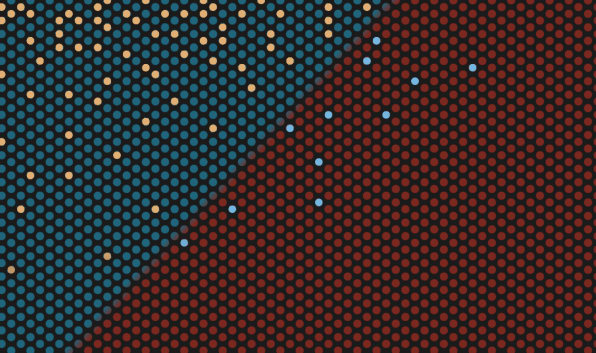
Another leader in this space is Netflix and its recommendation system, one of the oldest, most sophisticated, and lucrative AI in the market today. In 2000, Netflix introduced an algorithm that asked, “If you liked this movie, then you might want to watch that movie.” AI wasn’t such a buzzword back then, but Netflix needed to find ways to improve its customer experience over competitors, like brick-and-mortar movie rental stores. Netflix understood that people were more likely to rent movies if they could easily discover ones they might enjoy, and AI was used as part of the solution to this discovery problem.
Spotify exposes millions of us to personalized, algorithmically generated lists of music based on what we’ve listened to before, striking a careful balance between serendipity and relevance. It’s an excellent solution to a real business problem: as a freemium app, Spotify’s billion-dollar business depends on getting free users to start paying them $10 every month. So they’re asking themselves: What’s the fastest way to get users experiencing their core value— the ability to listen to whatever music they want, when they want it? Some companies might tackle this problem by revamping their UI and onboarding, or offering steep discounts. Spotify launched Discovery to unlock this problem.
Slack, Netflix, and Spotify epitomize the ability for companies to think big and smart small. Their use of AI isn’t showy or boastful; it’s helpful, appropriately used, and almost imperceptible to their customers. It helps them manage the onslaught of information online, or discover serendipitously. It boosts their productivity by making tasks easier or by taking over mundane day-to-day work.
This may be a less exciting concept to market or to tell the press. But the best way to leverage AI technologies is to see it for what it is, and pair that with a product-first mind-set. In other words, don’t think of AI as the solution to an unknown problem, think of it as a tool to help you solve a known one. Before adding AI to your product, spend plenty of time trying to answer these questions:
- What’s the problem at hand? Is AI the best way to solve it? If you’re trying to simplify a user interface, could that be done by allowing your customers to do it themselves? If you’re trying to predict response time between business and customer, could a simple calculated average do as well as a machine-learning prediction engine?
- What’s the risk of layering AI into your product? What’s the technical debt? How well do you understand the mechanisms behind the AI tool you might employ?
Once you’ve decided that AI is not right for your product, think big and start small. Most of us don’t have the oceans of data that Google and Microsoft possess, and that data is important to breakthrough research in this area. But we can all think about making small iterations, getting customer feedback, and improving from there. Companies may brag about using the most cutting-edge deep-learning algorithm in their products, but if their customers aren’t using it, is it even real?
–
This article first appeared in www.fastcodesign.com
Seeking to build and grow your brand using the force of consumer insight, strategic foresight, creative disruption and technology prowess? Talk to us at +9714 3867728 or mail: info@groupisd.com or visit www.groupisd.com